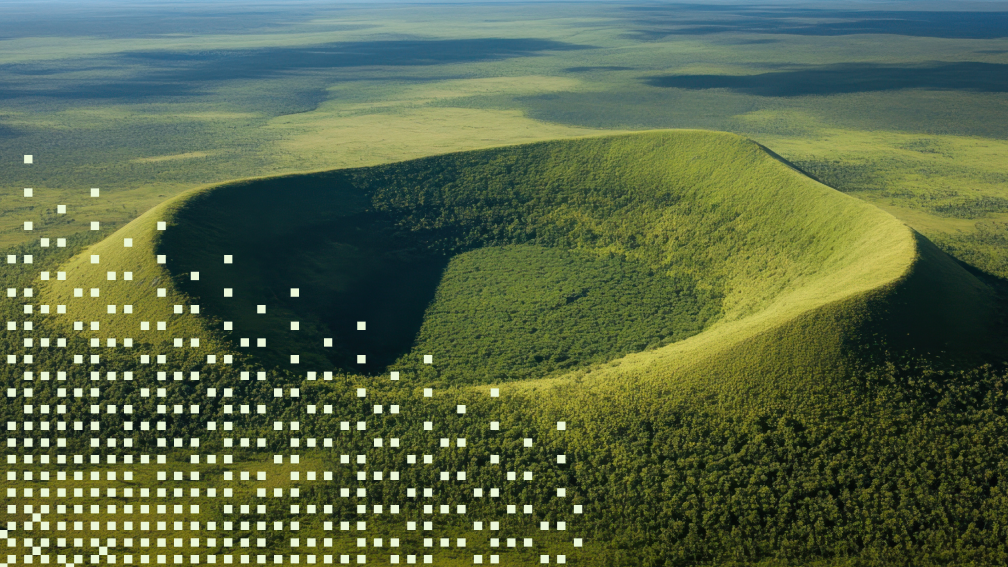
November 26, 2024 • 7 min read
Demystifying AI: Unlock Transformative Potential With Responsible AI Adoption
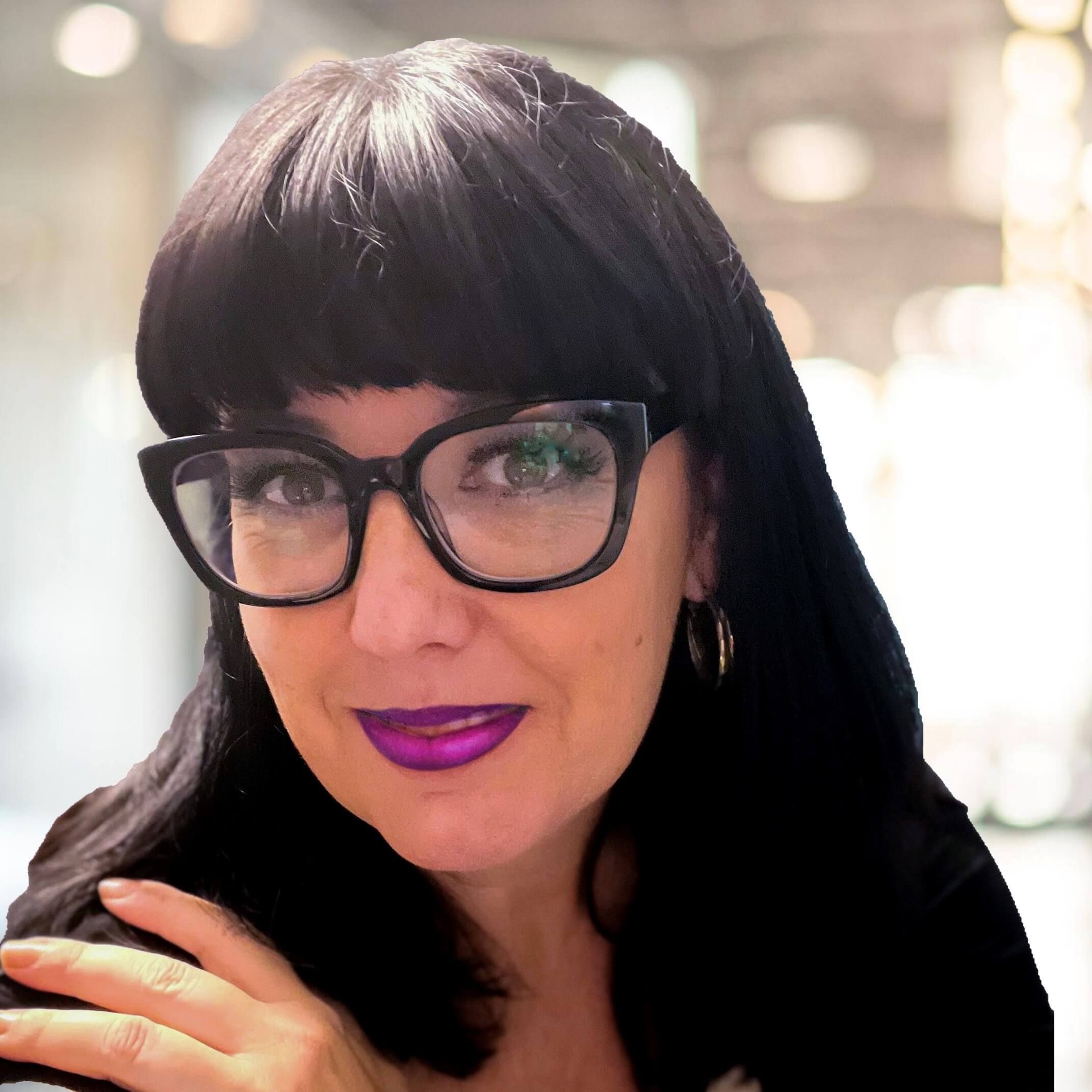
Melissa Pici
We’re almost 100 years from the first time artificial intelligence (AI) was depicted on screen in the German expressionist science fiction film Metropolis. AI has come a long way since then, and today promises to amplify capabilities and drive innovation. However, concerns over risks and unintended consequences often hinder its adoption. Businesses can pragmatically navigate the AI journey by better understanding the technology and what it can do, assessing the top risks, and creating a successful adoption strategy using industry best practices.
A Brief History of AI
Here’s a brief timeline of how AI has developed over time:
- 1950s: The term “artificial intelligence” is coined.
- 1960s/70s: An AI boom hits, with AI laboratories established worldwide. ELI A became one of the early natural language processing computers developed to explore communication between humans and machines. Waseda University in Japan created the WBO, the first full-scale humanoid intelligent robot that could walk, communicate, and grip and transport objects with its hands.
- 1980s: IBM created its deep blue computer, which beat chess champion Gary Kasparov.
- 2010s: The IBM Watson computer bested Brad Rudder and Ken Jennings at Jeopardy. Apple introduced Siri, Amazon Echo launched Alexa, Microsoft launched Cortana, and Google launched its Assistant.
- 2020s: OpenAI unveiled ChatGPT, a generative AI program that can produce text, images, audio, and more. In the first five days, 1 million users signed up.
What is AI, really?
AI is a technology that helps computers mimic human intelligence and problem-solving capabilities. It involves creating algorithms and models that learn from data, recognize its patterns, and make informed decisions. However, AI has different components, and there are also different AI types.
Coding is essential in implementing algorithms and fine-tuning models to achieve optimal performance. Developers can adjust parameters, experiment with various approaches, and improve AI systems through coding. They can also customize these solutions to specific tasks and industries.
Machine learning algorithms underpin AI with two types of learning:
- Supervised learning uses labeled data sets to determine the expected output and input, such as the probability of a customer churning.
- Unsupervised learning uses unlabeled data to discover patterns and helps solve clustering or association problems. It includes reinforcement learning, where the computer learns structures through rewards for desired behavior and punishments for undesired behavior.
Neural networks represent ML algorithms based on the human brain. Cells and nodes are connected, each processing inputs and producing an output sent to other neurons. Labeled data moves through the nodes or cells, and each cell performs a different function.
Deep learning ML processes inputs using a biologically based neural network design. This layered network can process massive amounts of data and pinpoint the weight of each link.
Generative AI, which powers ChatGPT, is a subset of deep learning models that can generate content, design art, software, and language translation outside of natural language processing—another type of AI in which computers can listen to humans talk and then analyze and give answers.
Computer vision allows computers to see like humans, combining many applications and sensing devices for practical cases.
Risky business or rewarding opportunity?
Of course, any technology is imperfect and, as a result, AI poses risks and challenges to businesses, such as:
- Accuracy: How will you know the data is accurate if you use AI to deliver information?
- Accountability: To date, AI has primarily been the purview of data scientists, but as more teams get their hands on it, how do they know to use AI ethically and appropriately?
- Intellectual property and copyright: If you use AI trained on copyrighted information, you might face severe fines and legal ramifications.
However, this doesn’t mean businesses should avoid AI altogether. It can benefit your audit, risk, or compliance program today. Companies must balance the risks with the rewards.
For instance, AI can help with tasks requiring much time but little brain power. From an auditor’s perspective, it can help me create an issue description or connect different controls to risks instead of doing a mapping exercise. AI can help improve production processes, search engine optimization, and process automation, such as pulling large amounts of data or looking for patterns between them.
How to get started with AI
If you’re interested in exploring how AI can benefit your business, here are a few quick tips:
- Understand what AI is and isn’t.
- Assess your readiness for adoption.
- Create an AI adoption strategy.
- Conduct a risk assessment.
- Create a cross-functional committee.
- Enlist a champion to help evangelize the AI opportunity.
- If you use a third-party AI app, look at the SOC 2 reports and try a pilot program.
We’re far past the point of ignoring AI—enough people want to use it. The best thing for your company to do is explore the opportunities and risks so you can drive responsible AI integration across the organization and capitalize on its transformative potential.
About the authors
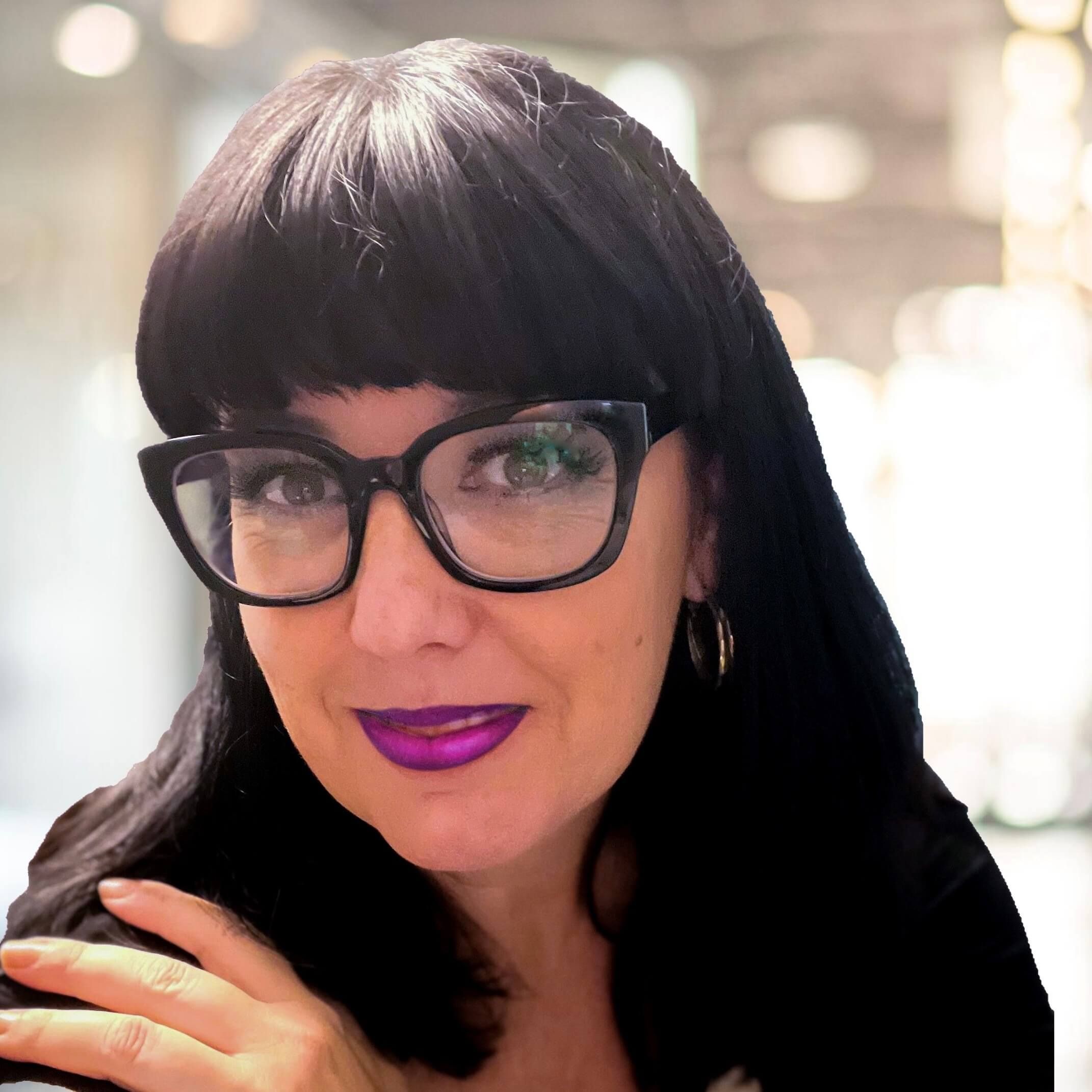
Melissa Pici serves as the Senior IT Audit Manager at Syniverse, having joined the organization over 19 years ago. In her role with the multinational organization, she works with cross-functional teams including Finance, IT, GRC, and Operations aiding them in understanding the criticality of a risk and controls-based foundation and the interconnectedness in everyday operations. Melissa manages and drives the annual SOC/ICFR audits, as well as designs and executes risk-based operational audits providing further insight to executive management in making risk-based decisions for the business.
You may also like to read
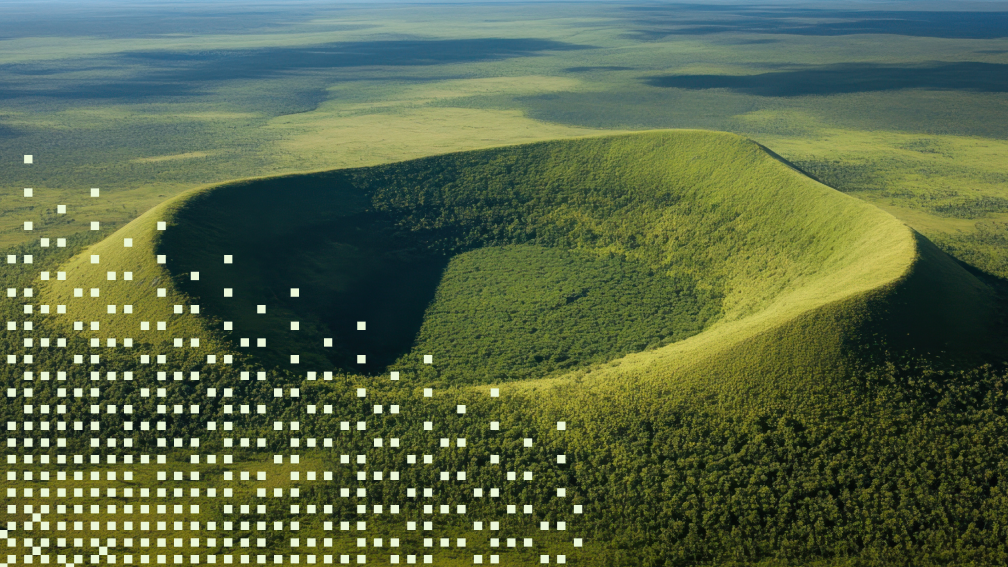
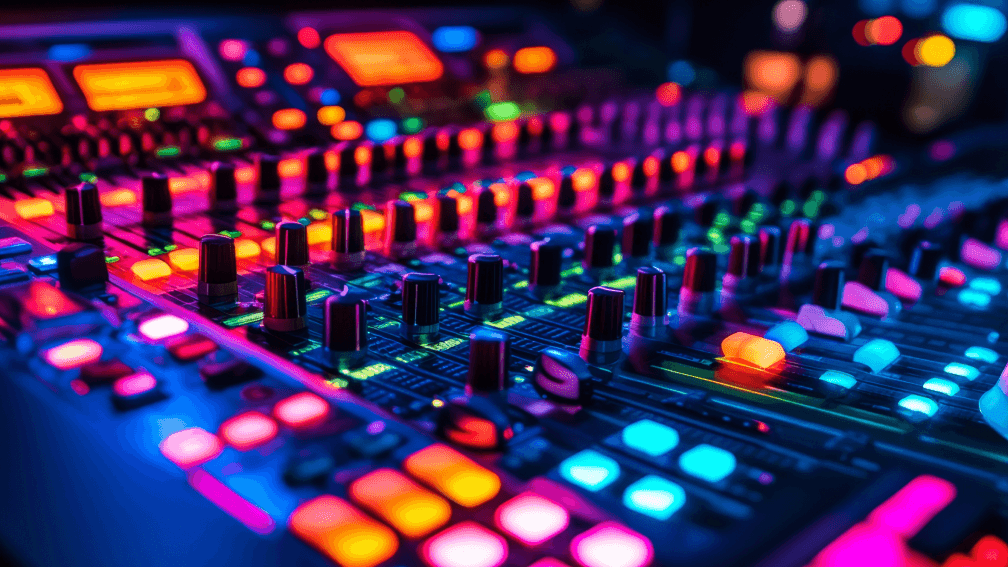
Commanding Compliance: Demystify the Common Control Set
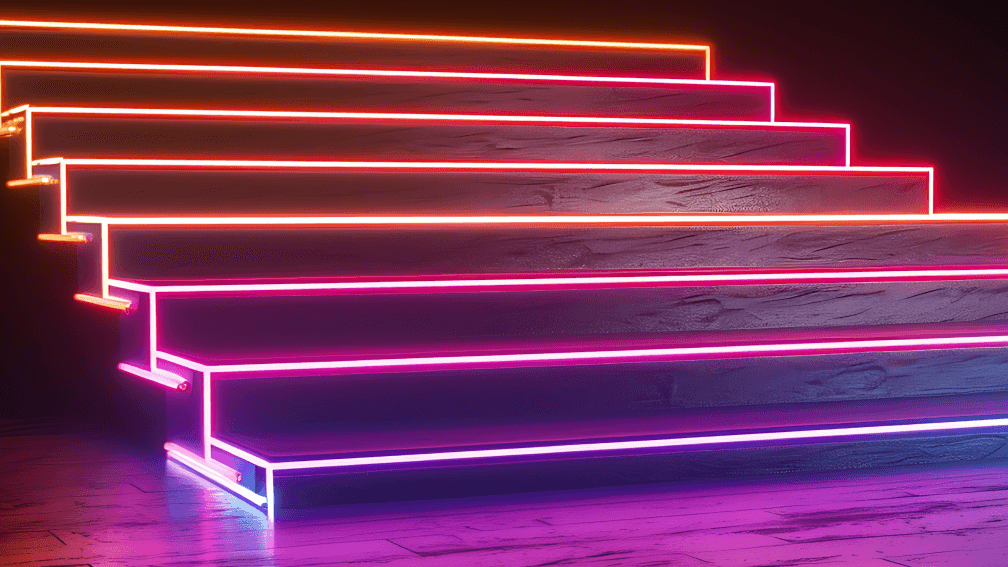
Practical Steps for Applying NIST CSF 2.0 to Third-Party Risk Management
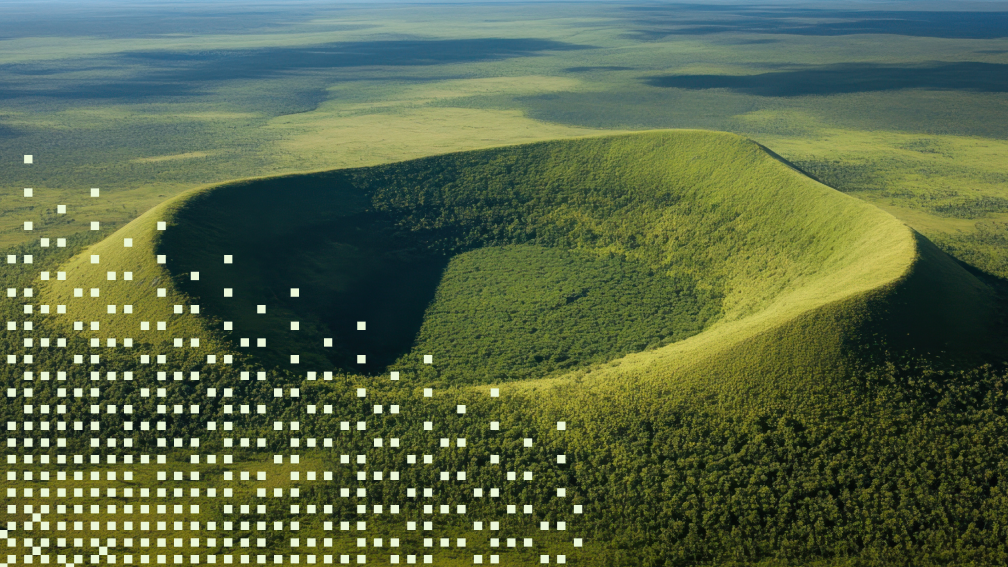
AI Governance: Automated Control Testing for ITRC
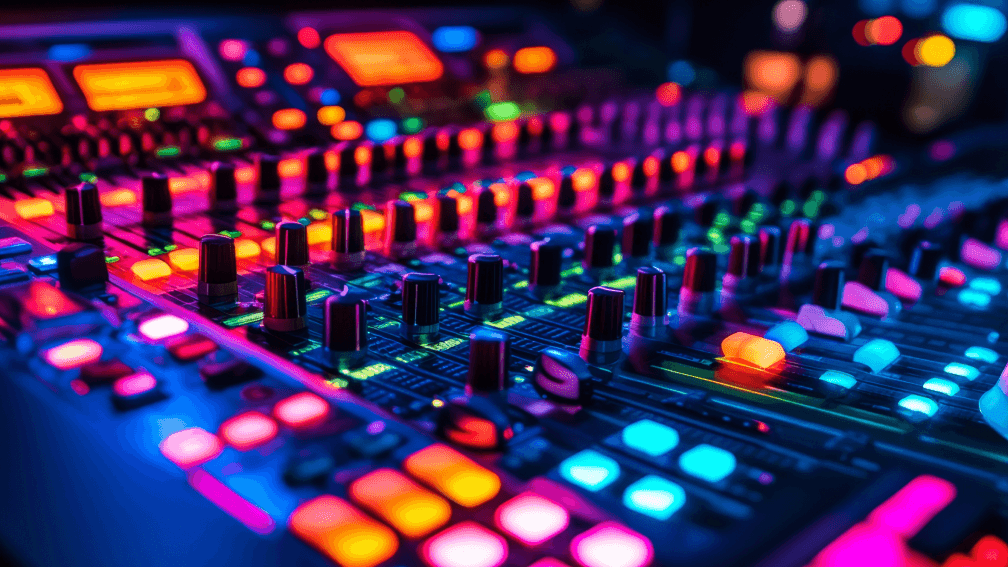
Commanding Compliance: Demystify the Common Control Set
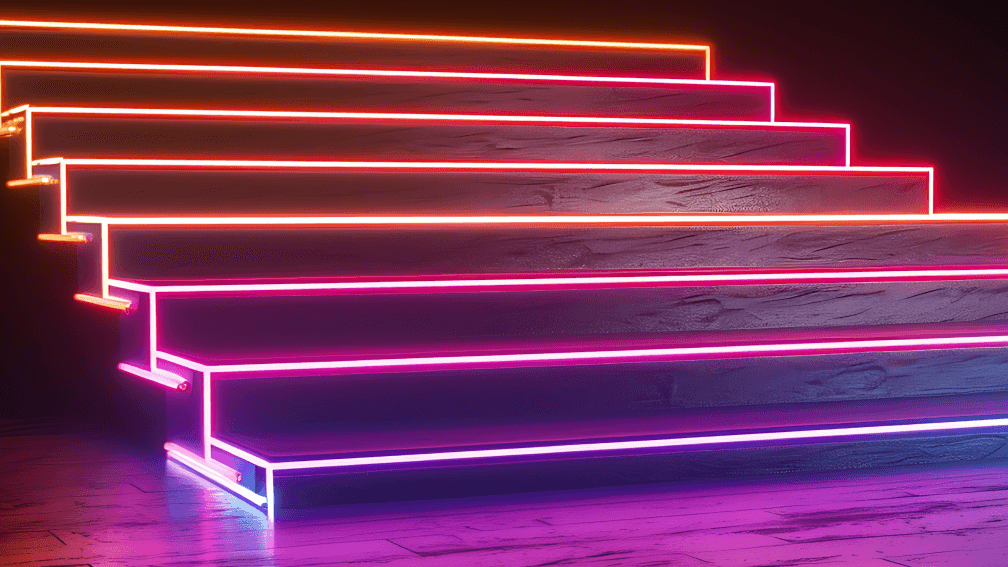
Practical Steps for Applying NIST CSF 2.0 to Third-Party Risk Management
Discover why industry leaders choose AuditBoard
SCHEDULE A DEMO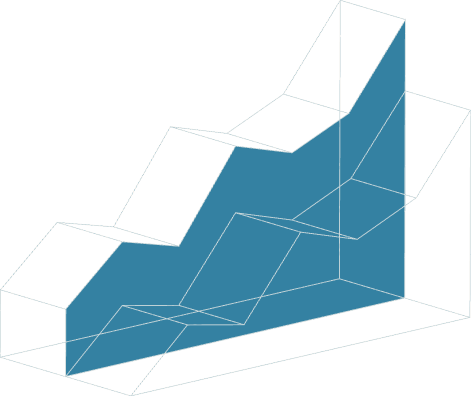
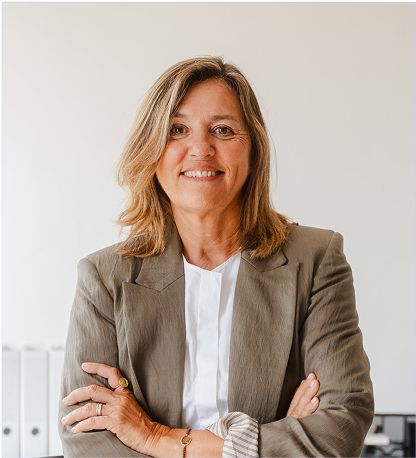