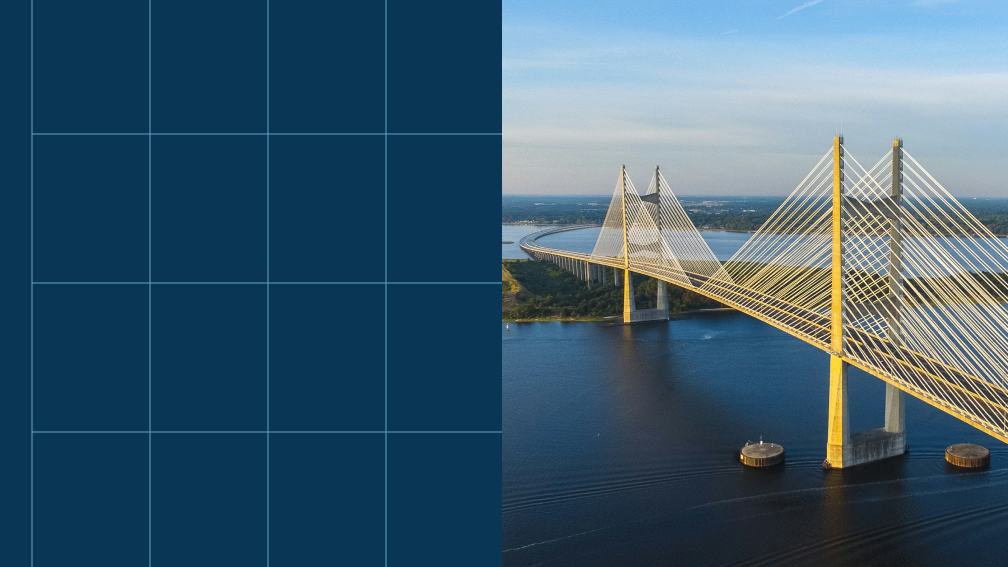
April 9, 2025 • 11 min read
AI Governance: Automated Control Testing for ITRC
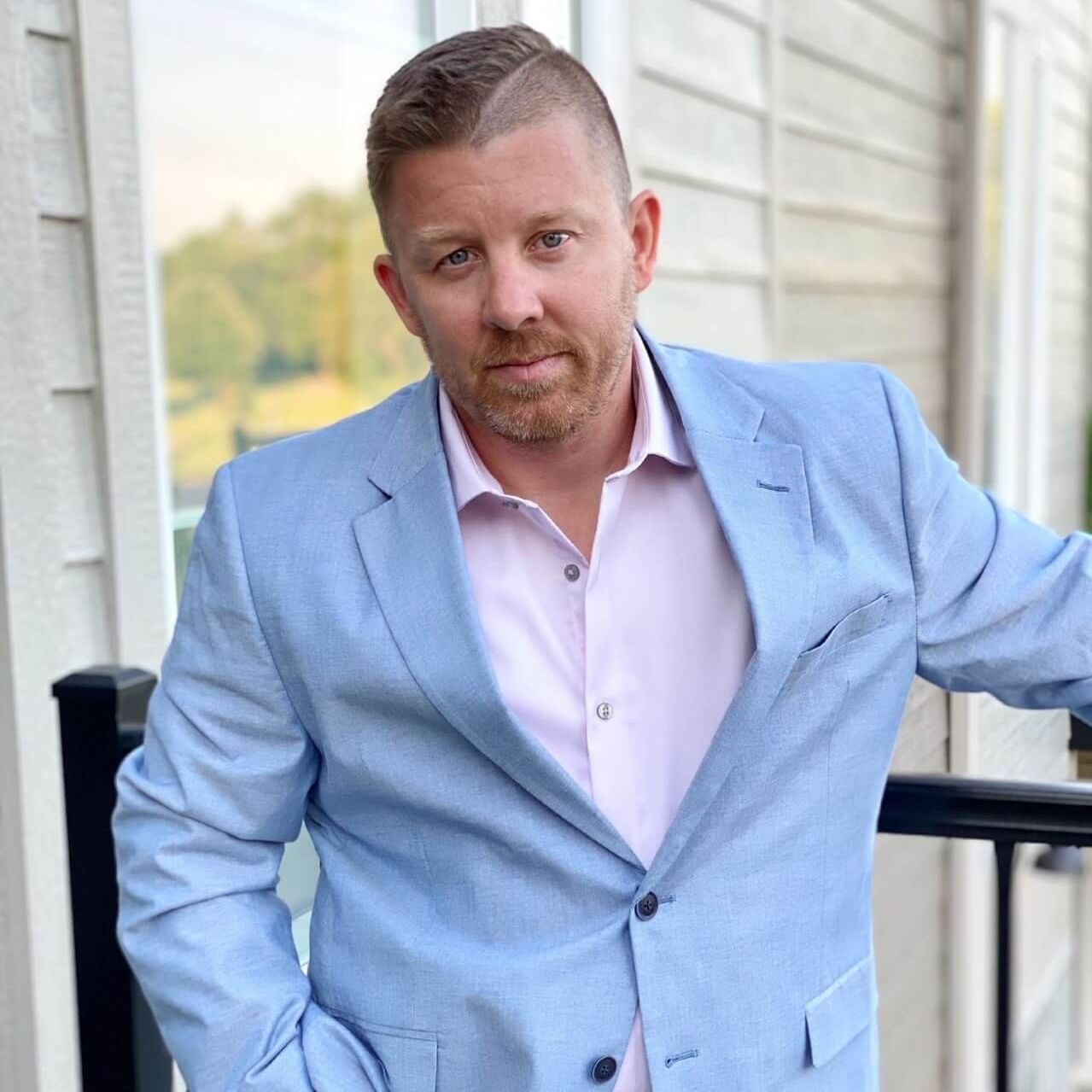
Mike Miller
As Artificial Intelligence (AI) continues to reshape the technological landscape, its integration into governance and compliance processes is not just innovative, it’s essential. For organizations navigating the complexities of regulatory compliance, particularly in frameworks like the Information Technology Risk Controls (ITRC), automation through AI presents a game-changing opportunity. In this blog, we explore how automated control testing, driven by AI, supports robust governance in the ITRC context and helps organizations future-proof their compliance strategies.
The Rise of AI in Governance
AI governance refers to the frameworks, policies, and practices that ensure the responsible development, deployment, and oversight of AI systems. As organizations increasingly leverage AI for decision-making and operational efficiency, the importance of transparent, ethical, and auditable AI use becomes paramount.
Governance in this context also extends to how AI is applied to support enterprise risk management and compliance. One of the most promising areas where AI is making a significant impact is in automated control testing—especially within the realm of ITRC.
Understanding ITRC: A Foundation for Risk Management
The Information Technology Risk Controls (ITRC) framework provides a structured set of controls aimed at identifying, managing, and mitigating technology-related risks. It includes policies, processes, and technical safeguards designed to protect data, systems, and IT operations from threats.
Traditional methods for testing these controls often involve manual audits, sample testing, and interviews—methods that are time-consuming, reactive, and prone to human error. As digital infrastructure becomes more complex and compliance requirements evolve, the limitations of manual control testing become more apparent.
Enter automation.
Automated Control Testing: What It Means
Automated control testing involves the use of technology to continuously monitor and evaluate the effectiveness of internal controls. Rather than relying on periodic manual reviews, organizations can deploy scripts, bots, and AI algorithms to check if controls are in place and functioning as intended.
With the integration of AI, this automation becomes even more intelligent. In the ITRC context, this means faster, more accurate, and more consistent assessments of control environments. AI can:
- Analyze vast data sets at scale
- Detect anomalies and potential control failures in real time
- Predict risk exposure based on patterns and trends
- Learn from historical control performance to suggest improvements
The Role of AI in Control Testing
Let’s break down how AI enhances the process of control testing for ITRC:
1. Data Aggregation and Normalization
AI algorithms can pull data from multiple sources—logs, tickets, change management systems, vulnerability scanners—and standardize it for analysis. This helps create a unified view of the control environment, eliminating silos and reducing blind spots.
2. Natural Language Processing (NLP) for Policy Review
NLP can read and interpret control documentation, policies, and audit logs. This allows for automated mapping of controls to regulatory requirements and helps in identifying outdated or missing controls.
3. Anomaly Detection
Machine learning models can establish baselines for what “normal” looks like in system behaviors or control performance. They can then flag deviations that may indicate control failures or compliance breaches.
4. Predictive Analytics
AI can anticipate control failures based on past data, offering insights into potential vulnerabilities before they become compliance issues. For example, if patching timelines are frequently missed in a particular business unit, AI can identify this trend and alert stakeholders proactively.
5. Automated Evidence Collection
For audit readiness, AI systems can automatically gather and log evidence of control effectiveness, such as access logs, encryption statuses, or configuration snapshots. This reduces the burden on teams during audits and enhances transparency.
Benefits of AI-Driven Control Testing
The advantages of applying AI to automated control testing for ITRC are significant:
- Speed: Continuous, real-time testing far outpaces periodic manual reviews.
- Scalability: AI systems can handle complex, distributed IT environments with ease.
- Accuracy: Reduced human error means more reliable results.
- Efficiency: Automation reduces the manual workload and frees up teams for higher-value tasks.
- Proactiveness: Predictive insights help address issues before they become costly problems.
- Audit Readiness: Real-time evidence collection ensures that audit trails are always complete and up-to-date.
Real-World Example: AI in Action
A real-life example from our industry was a multinational enterprise subject to GDPR, SOX, and ISO 27001. Its IT environment spans multiple data centers and cloud platforms. Traditionally, compliance audits involved labor-intensive manual reviews of access controls, patch management, and incident response logs.
By integrating AI-powered control testing tools:
- The organization deployed agents to continuously monitor access logs, flagging any unauthorized or unusual access attempts.
- NLP algorithms reviewed policy documents to ensure that procedures aligned with regulatory expectations.
- Predictive models highlighted systems at risk of failing controls—such as delayed patching or outdated configurations—prompting preemptive action.
As a result, the company saw a 60% reduction in audit preparation time, a 40% improvement in control effectiveness, and significantly reduced risk exposure.
Challenges and Considerations
While the benefits are compelling, adopting AI for control testing isn’t without its challenges:
1. Data Quality
AI is only as good as the data it processes. Poor data quality or incomplete datasets can lead to inaccurate insights.
2. Model Transparency
Many AI models, especially deep learning systems, operate as “black boxes.” For governance and compliance, explainability is crucial. Organizations must choose models that offer traceable logic or leverage explainable AI (XAI) techniques.
3. Change Management
Automating control testing represents a significant shift. Stakeholders must be educated and trained to trust and use AI outputs effectively.
4. Integration Complexity
Deploying AI across existing legacy systems may require significant integration efforts. The ROI, however, often justifies the upfront investment.
5. Regulatory Acceptance
Some regulators may be cautious about fully automated compliance testing. Organizations should maintain transparency and human oversight, especially in critical control areas.
Best Practices for Implementing AI in ITRC Testing
To make the most of AI for control testing in the ITRC framework, consider these best practices:
- Start Small: Begin with a pilot project in a non-critical control area to demonstrate value and fine-tune the system.
- Ensure Explainability: Use models and tools that clearly justify their decisions.
- Collaborate Across Teams: Involve risk, compliance, security, and IT teams in implementation planning.
- Maintain Human Oversight: AI should augment, not replace, human judgment.
- Monitor and Tune Continuously: AI models need regular tuning based on feedback and changing conditions like any system.
The Future: Toward Continuous Compliance
As AI matures, it will push organizations closer to the goal of continuous compliance—a state where control testing and risk monitoring happen in real-time, seamlessly integrated into day-to-day operations. This paradigm shift redefines compliance from a periodic obligation to a dynamic, ongoing capability.
When enhanced by AI, ITRC frameworks move beyond static checklists into living, adaptive systems that evolve with the organization and its environment.
Conclusion
AI-driven automated control testing is not a futuristic concept—it’s a present-day enabler of smarter governance. In the context of ITRC, AI offers a path to stronger controls, improved efficiency, and a more proactive risk posture.
As regulatory expectations increase and digital complexity grows, organizations that embrace AI for governance will be better positioned to thrive. The key lies in combining cutting-edge technology with strong oversight, ethical practices, and a culture prioritizing accountability.
AI is not just transforming how we test controls—it’s also transforming how we think about trust, compliance, and governance.
About the authors
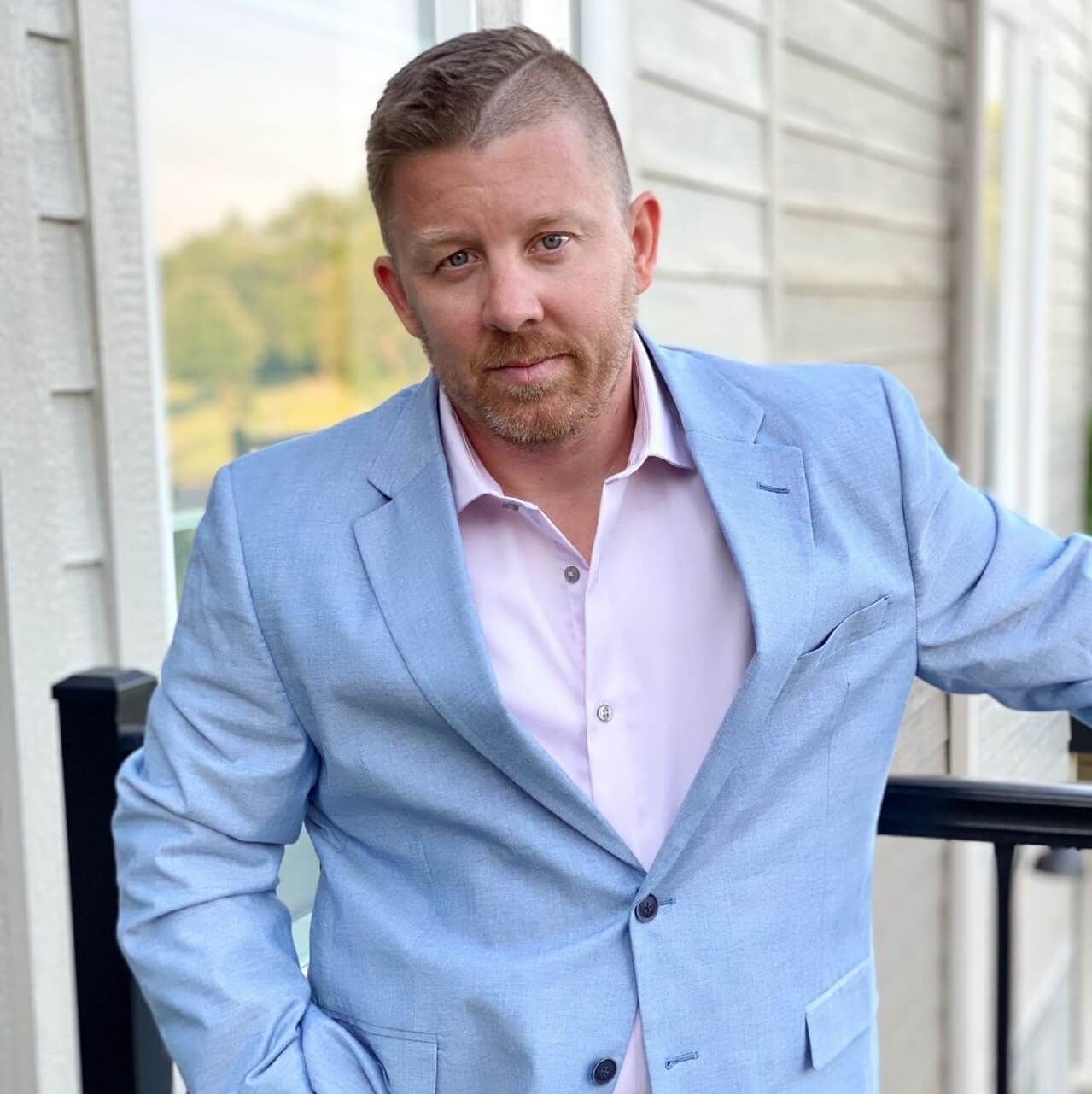
Mike Miller is a vCISO at Appalachia Technologies and is a 25+ year professional in Tech and Cyber Security. Connect with Mike on LinkedIn.
You may also like to read
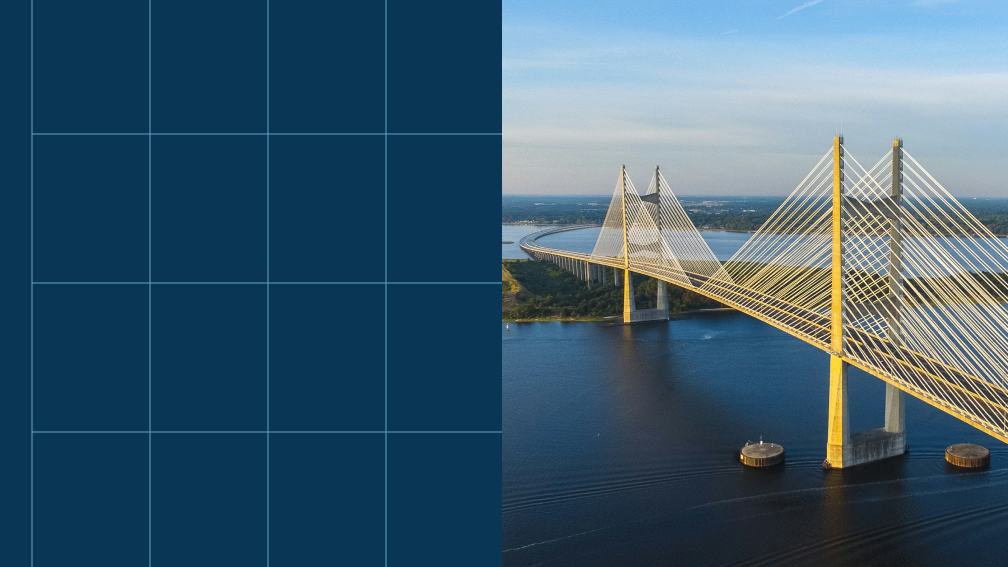
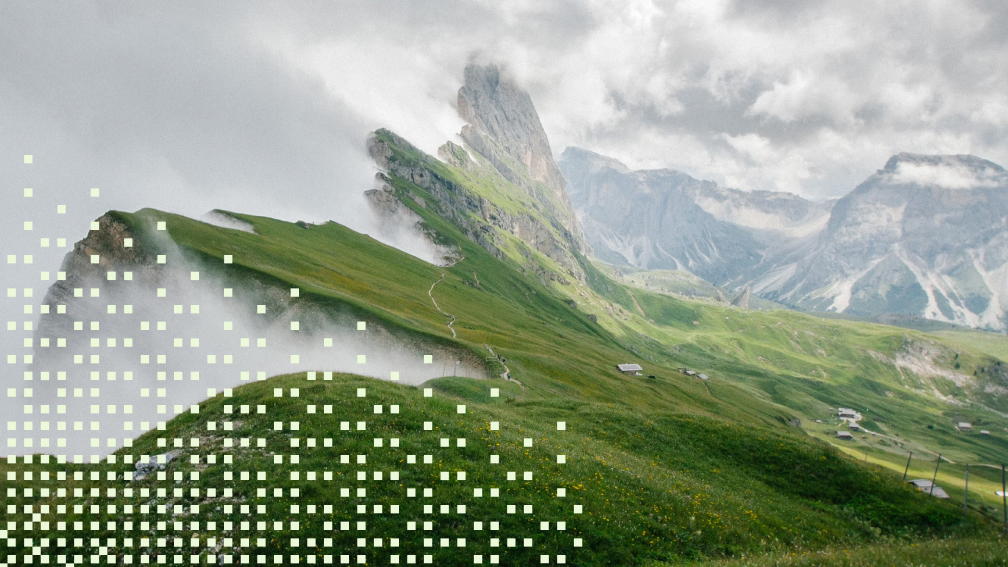
GRC automation: What finally works for audit, risk, and compliance
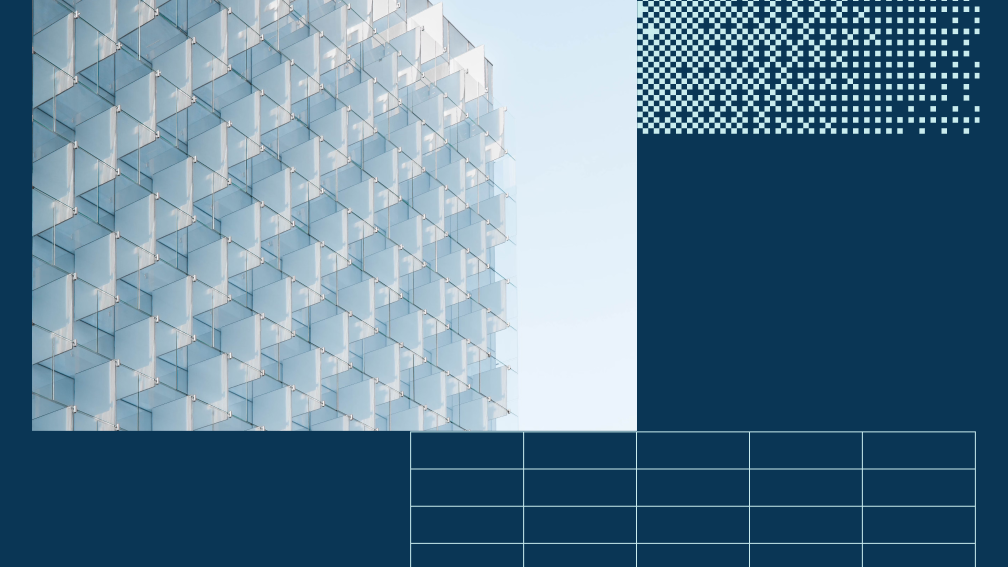
Security log retention: Best practices and compliance guide
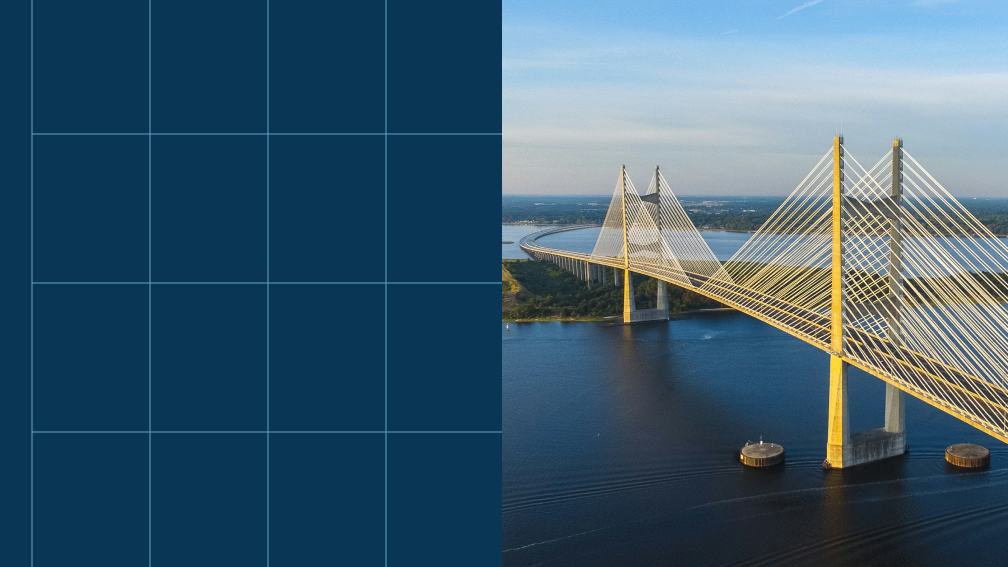
Cybersecurity GRC for proactive risk and real-time visibility
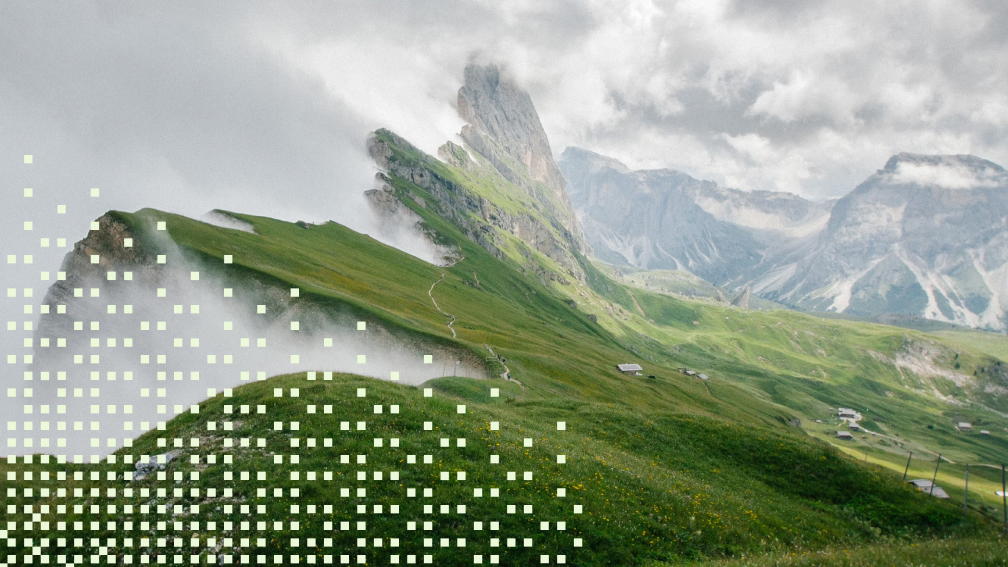
GRC automation: What finally works for audit, risk, and compliance
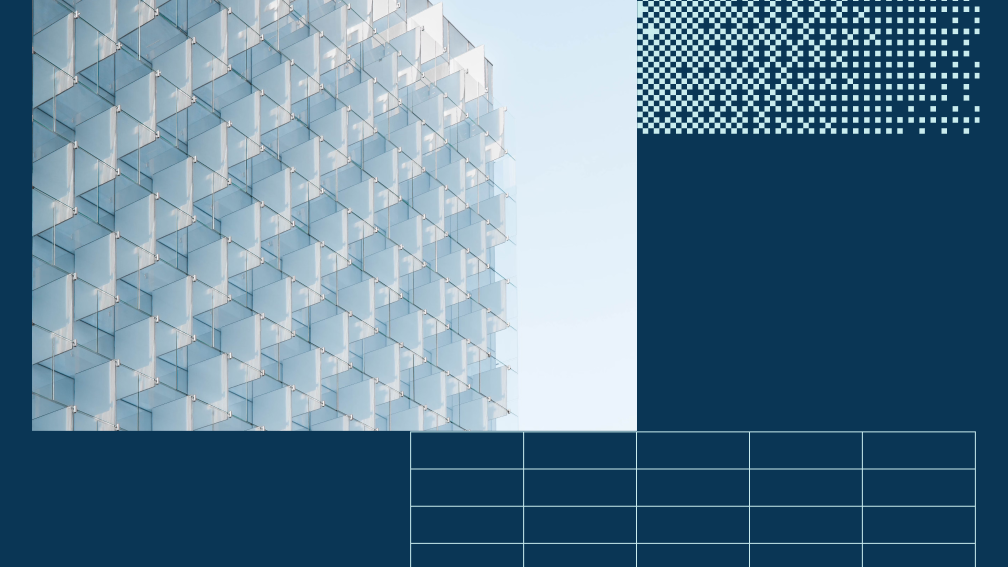
Security log retention: Best practices and compliance guide
Discover why industry leaders choose AuditBoard
SCHEDULE A DEMO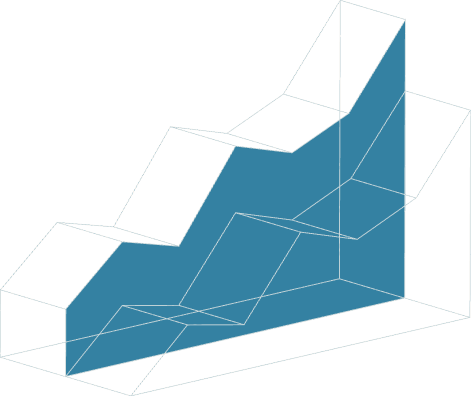
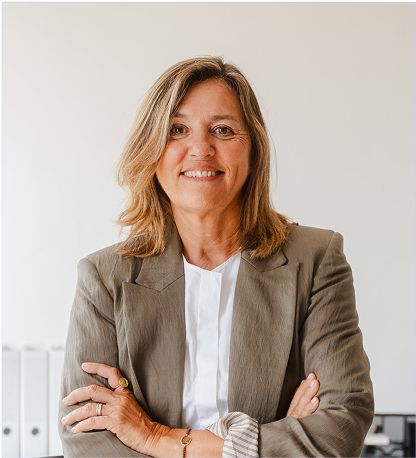